In practice, the barrier to impact can often be traced back to a focus on building algorithms before identifying appropriate use cases for the output. That leads to elegant tech solutions chasing problems that don’t exist or don’t deliver tangible value to the business. At the same time, end users often get overwhelmed by the extensive new toolsets and data that they were not trained to use. Picking the right use case and enabling the organization is just as important as writing good algorithms, and it should come first in the solution-design process.
A Success Story
What does a best-practice case look like? A global distributor of spare parts, whose core business depends on efficient inventory management, started by identifying forecasting use cases. If ML were to raise its forecasting accuracy, it could both improve customer service levels and reduce costly overstock. After a quick and iterative pilot, it became apparent that ML could indeed deliver against these use cases for several large customer accounts. Thus, the distributor proceeded to build up both a production-forecasting solution and a data-science team able to maintain and integrate the new tool throughout business processes. Within six months, the organization had developed a completely new capability that delivered against the value identified in the pilot.
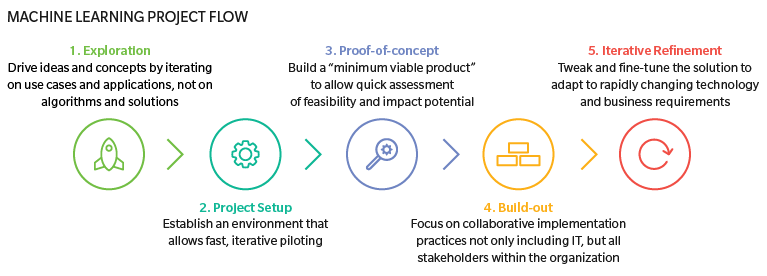
This implementation was a success because the focus from the beginning was on ‘how will we use this’ and ‘what tools, organizational construct, processes, and governance are needed to make this work’? Clear use cases and capability assessment preceded the actual solution build out and implementation. The distributor realized early on that a forecast that was 80 percent accurate supported by working processes would prove more valuable to the business than a forecast that was 95 percent accurate without the proper organization and processes to make good use of the predictions.
In the end, the company was happy both with its forecasting product and the new capabilities it had developed. Because business users had always been included as an integral part of the development process, the data-science team enjoyed a high standing throughout the whole organization. This same team has thereafter become the go-to for any analytics or data-automation question and launched multiple new projects within the organization.
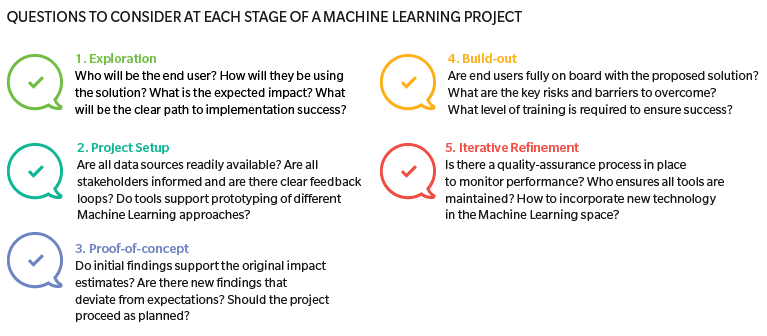
In this example, one successful ML use case has essentially challenged a whole organization—to both buy into a new tool, and to build a team driving automation and data science throughout all aspects of their business.
By itself, ML is a group of algorithms that thrives on voluminous amounts of data. But to unlock true impact in business contexts, the algorithms need to be tailored in a joint and inclusive effort, starting with a clearly defined use case and tangible plan for how interlocking business processes will integrate and deliver benefit in the end.