Our recent article on overhauling bank credit infrastructure underscored the critical importance of cleaning up “dirty” data. You can read it here.
Despite unremitting regulatory pressure and millions of dollars of investment in data governance, many banks have made little real progress in addressing the root causes of dirty credit data. The consequences of this failure are enormous; data quality issues undermine basic credit risk management. For example, without reliable data like single client identifiers or product codes, banks struggle to connect various exposures in different products to the same counterparty. Without timely delivery of critical data like mark-to-market collateral values, net exposure calculations may be incorrect. Indeed, poor data often means that credit officers spend more time debating the accuracy of underlying data than they do addressing the critical credit issues that the data should inform. At a large bank, we found credit officers spent on average one to two hours every day checking and remediating exposure and collateral data.
The Covid-19 crisis has served as a wake-up call for risk departments, underscoring the urgent need for better credit data. In the absence of clean data, credit officers have scrambled to answer critical questions about primary exposures to vulnerable industries such as airline, retail, and oil and gas companies. Many have been at a loss to explain potential secondary exposures to affected industries via supply chains or investment funds. Others have struggled to track drawdown behaviour across products at client level, connecting the dots only after a considerable delay.
The crisis has shown how banks with better credit risk data can see potential problems earlier and react more quickly, getting ahead of peers with poor data. They can also operate more efficiently, dispensing with legions of “data elves” that manually validate and clean dirty data.
Moreover, the benefits of clean credit data have not been lost on regulators. Even before the current crisis, many regulators had become more proactive in pushing banks to show credible plans for addressing the root causes of credit data issues. Amidst continuing economic uncertainty, banks need to be able to quickly respond when the next spasm strikes. Regulators are watching. Indeed, the ECB’s recent letter to Significant Institutions underscores their concern about banks’ ability to manage distressed portfolios.
Why so dirty?
Recent efforts to clean up data, starting with the BCBS 239 programmes kicked off in the wake of the financial crisis, have not led to any appreciable improvement in overall data quality. Why?
First, many banks have focused on putting in place the foundational elements of data governance in an ivory tower and ignored the “data elves” at work in the coal face. Data ontologies, taxonomies, ownership grids, and policies are all important foundational elements of a good data governance strategy. But they are not worth much if they do not have direct impact on day-to-day data flows. Too often, this connection is missing.
Second, many banks do not have a solid operational approach for their critical data processes. At most banks, the lack of process-related KPI’s to track drivers of poor data (e.g. manual uploads, adjustments, hand-offs, checks, and controls) is revealing. Banks are not applying the same level of operational rigour to critical report generation processes that they apply to, say, mortgage or other key customer processes.
Third, the systems in place to ensure proper data “ingestion” into risk processing environments do not work well. In fact, traditional ETL (Extract-Transform-Load) tools have become a major cause of data indigestion! These systems struggle to control the quality of incoming data. For large banks, multiple layers of business rules (amounting to millions of lines of code) parse thousands of feeds per day from dozens – sometimes hundreds – of different data sources, creating an impenetrable barrier for those diagnosing the root causes of data issues.
Banks need to re-think their approach to cleaning up credit data as a matter of urgency. Based on practices at peers that are making progress, we have four recommendations for next generation credit data remediation.
Recommendation one: Join the gold rush
Leading banks are consolidating upstream data repositories to create single sources of truth or “golden sources” for critical types of data. Source consolidation reduces the effort that Risk (and other departments) need to expend in data ingestion by decreasing the number of feeds by as much as 75 percent. Building golden sources for reference data domains (for example, book, product, party, legal entities, instruments) is particularly beneficial because so much Risk data remediation work typically involves sorting through inconsistent reference data to create comparable data sets.
Leaders are also ensuring that the data in these “golden sources” are accurate, up-to-date, and complete, reducing the effort involved in downstream data clean-up by as much as 95 percent. Since “accurate” may have a different meaning for Risk than for data owners, a few banks have put in place SLAs to define specific data quality standards.
Recommendation two: Treat your data indigestion
Pioneer banks bank are replacing their existing, over-engineered ETL tools with modern data orchestration layers, often leveraging tools from cloud storage providers that are highly customizable and less expensive than traditional tools.
These orchestration layers are typically simpler, more efficient, and more flexible than older data ingestion systems. They contain far fewer business rules and have “user friendly” libraries that enable non-technical users to have a clear understanding of previously “hard-coded” adjustments.
Recommendation three: Measure, measure, measure… and implement a data “star chart”
Leading banks are also deploying incentives and penalties in order to encourage ownership and accountability amongst data providers. Corporate goodwill and good intentions are often not enough. Without carrots and sticks, it is tough to motivate busy executives to dedicate time and effort to clean data.
A few years ago, after a series of reporting issues, a leading European bank set up a central data quality team to monitor and control data inputs. This team flagged “dirty data” issues and communicated them back to data providers. If these issues persisted, the offending data providers received a punitive charge for internal reporting purposes until the problem was fixed. Senior executive bonuses could be directly affected by a failure to respond. After one year, the bank noticed a dramatic turnaround in its data quality, with errors and restatements falling by 80%. Moreover, the scheme created much greater awareness of (and cultural aversion to) dirty data.
Leading banks like the one mentioned above are measuring data quality with a level of precision that allows them to identify the root causes of problems. Ideally, these measurements should rely on controls and checks carried out at the source of data to give Risk and other users advanced warning of issues.
Recommendation four: Clean up the clean-up crews
Leading banks are revisiting the need for large teams of manual data fixers. Many risk departments have built clean-up crews to validate, to correct, and to enrich data. These armies of data fixers often constitute a cheap and expedient way to deal with dirty data. But the remedy can be worse than the disease over the long run. Clean-up crews reinforce a culture of manual workarounds that can become self-perpetuating and delay a proper reckoning with the root causes of dirty data.
Re-thinking credit data
Banks can no longer afford to ignore the knocking and rattling coming from their credit engines. Poor credit data – like contaminated fuel in a combustion engine – can undermine performance, make it difficult to keep up with competitors, and ultimately cause a complete breakdown. Against the backdrop of the current crisis, banks need to revisit their existing programmes and take decisive action to clean up credit data once and for all.
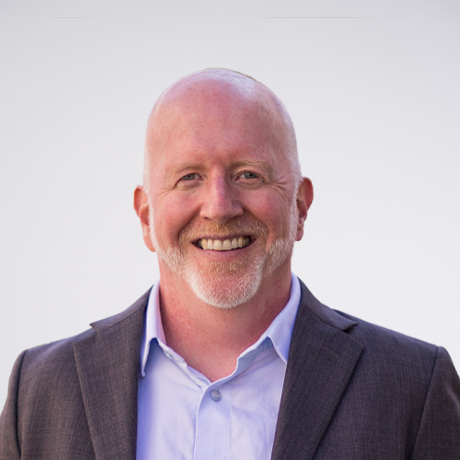
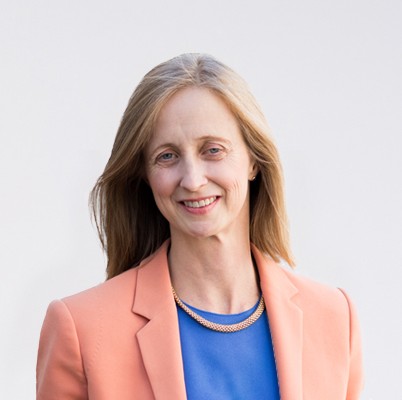
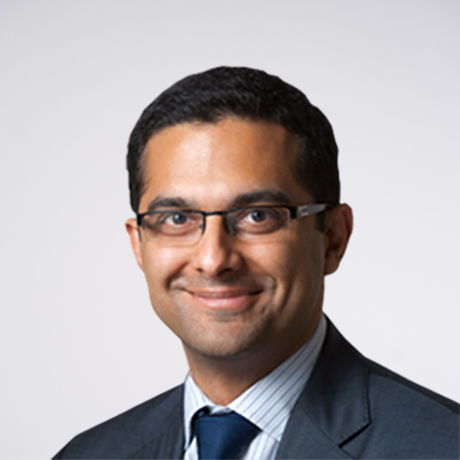
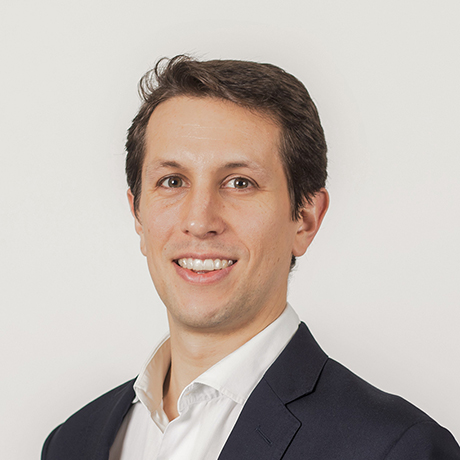