Start the Journey Now...
Pave the way, and establish a successful AI model management infrastruture
Financial services executives view artificial intelligence (AI) with a powerful mix of excitement and concern. With new proofs of concept, innovation labs and investments in technology appearing every day, financial institutions are eagerly exploring AI to improve business decisions, customer experiences and risk management outcomes. While AI promises great opportunities for financial services firms to work faster and smarter, it faces substantial scepticism among stakeholders, including bank regulators.
Our paper provides current AI use cases and how AI models differ from traditional approaches. We present seven paths that financial institutions need to start exploring now, in order to lay the foundation for addressing these concerns and enabling the new age of analytics. By starting down these paths, banks can establish a high-level framework within which this rapidly evolving and complex modelling can be addressed systematically and responsibly.
How AI is Different
Banks are implementing AI models in order to increase revenue or reduce cost through better and faster decision-making. Customer segmentation, fraud detection, price optimization, compliance monitoring, and loss forecasting are only a few examples of areas where financial institutions have built models using a range of approaches such as clustering algorithms, deep neural networks, and sentiment analysis.
Traditional Models vs. Next Generation AI Models
Over the past few decades, model management frameworks were designed around traditional models, and today, AI models present challenges for those existing frameworks. How would a model that is updated daily or even in real-time fit in this validation framework? It’s a significant effort with multiple layers to get to a state where model management frameworks are modernized to fully accommodate AI and other advanced analytics.
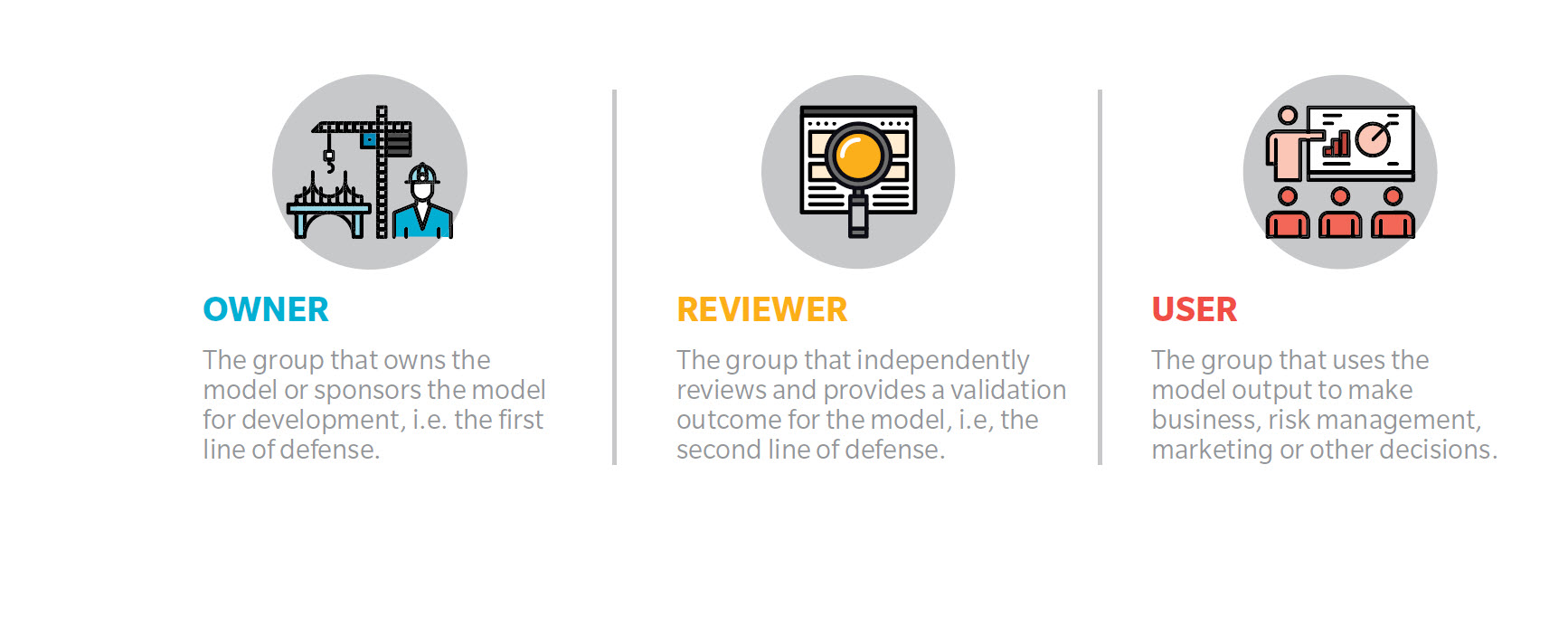
Institutions planning to invest in and implement advanced AI capabilities need to start adjusting their model management framework, and address the concerns of the three groups of stakeholders
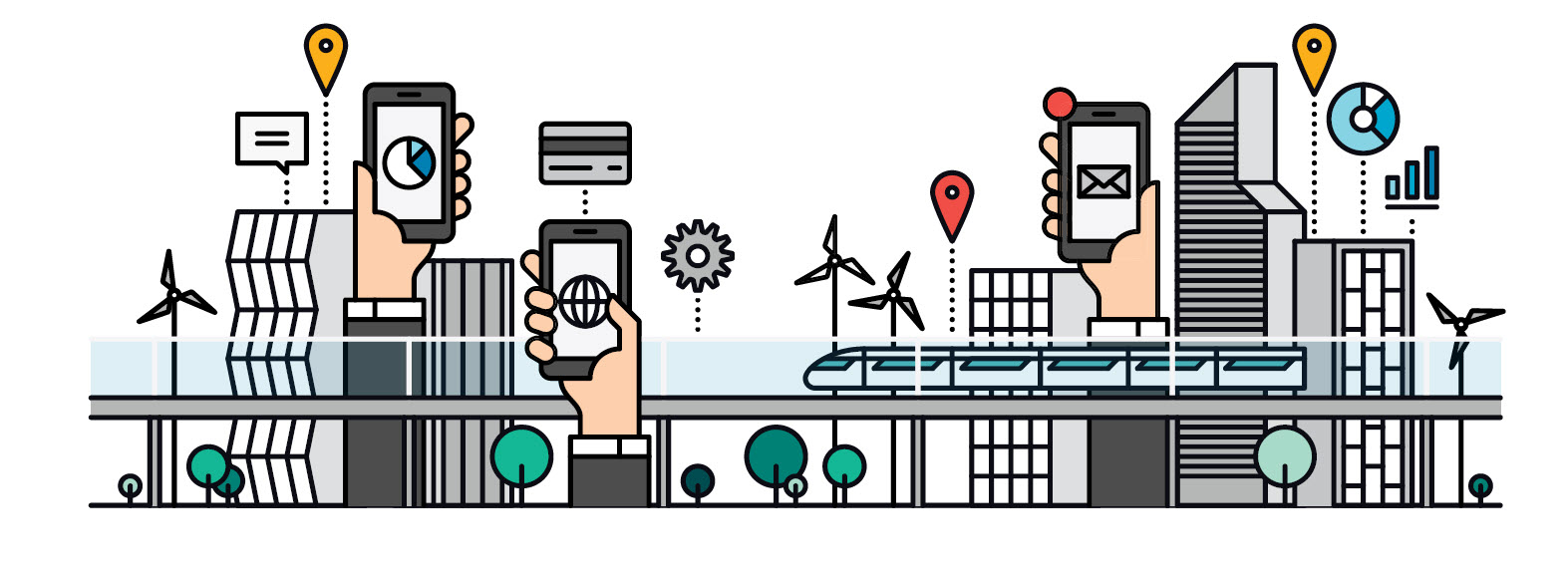
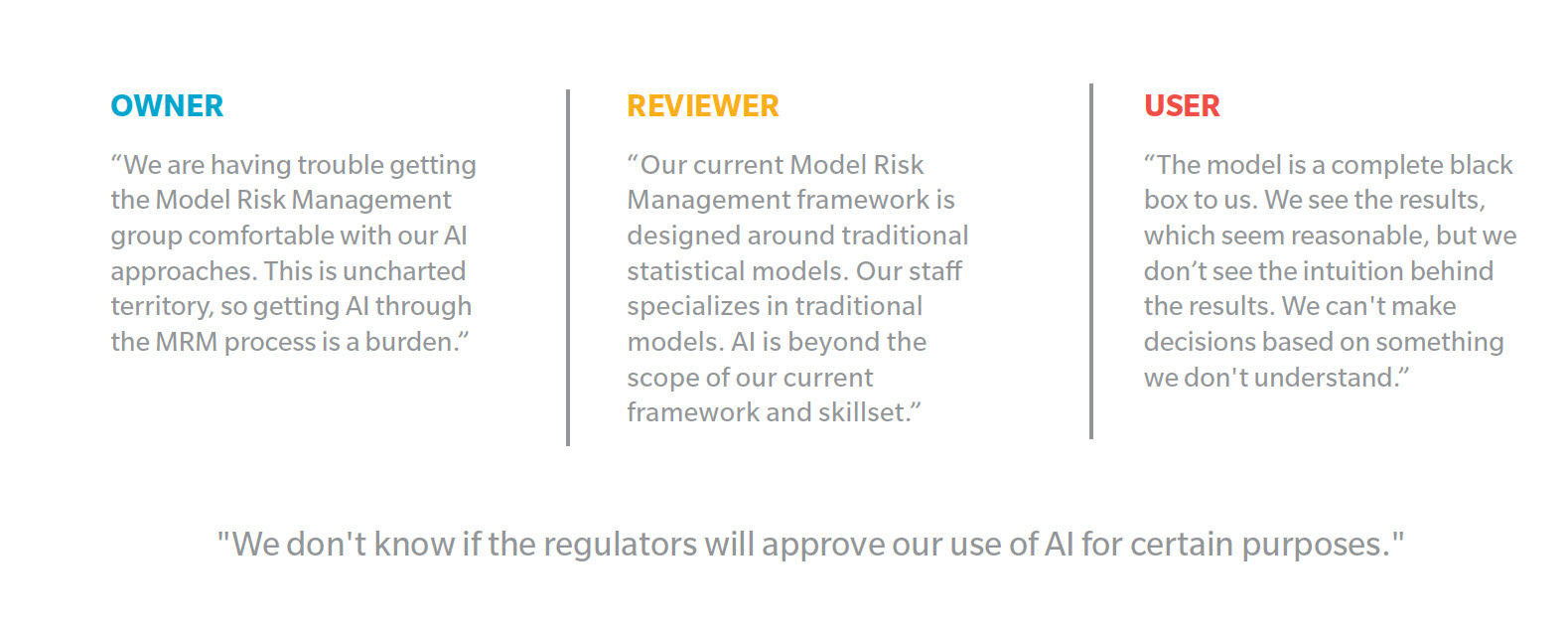
Move in the right direction, and enable AIThese concerns can have a tangible negative impact on a financial institution as investments in AI can be lost or cost the bank more time and resources while the model is forced through the traditional framework.
7 paths that financial institutions should start exploring
1. Stay at the boundary initially
Initially use AI to inform, or as an input to, traditional models as opposed to building a standalone AI model. For example, determine data segmentation based on AI and then develop traditional model using that segmentation.
2. Conduct parallel runs
Develop AI models as challengers to existing traditional models. Run models concurrently to understand and demonstrate relative strengths and weaknesses.3. Update the tiering framework
Designate AI as a distinct model type in model risk policy and formalize definitions. Incorporate AI into existing model-tiering framework and develop associated guidelines and procedures.
4. Focus on on-going monitoringFor AI, shift focus from regular validations to on-going monitoring as primary tool of model risk management. Heighten expectations and requirements for the Owner to develop robust on-going monitoring plans specific to AI.
5. Build the necessary skillset to own and review AI
Articulate the new technical, process and governance-related expertise and skills that will be required from the Owners and Reviewers as a result of using AI. Design training programs and hire new staff to fill any gaps. Consider establishing a group dedicated to AI within the independent review function.
6. Host Pilot User sessions
Identify Pilot Users that will collaborate with the Owners during and after development to inform and understand approach. Have Pilot Users organically spread the message and educate colleagues.
7. Understand the pros and cons
Focus on thoroughly understanding the strengths and weaknesses of a particular approach (e.g., through sensitivity analysis) instead of the full mechanics, which can be technically challenging. Design workshops and training to educate users.